What connects ChatGPT and antibiotics?
History has a curious answer: 1944. In that year, scientists proposed the first artificial neural network, a technology that later led to the birth of deep-learning and artificially intelligent systems like ChatGPT. The same year, biologists discovered streptomycin, the world’s first aminoglycoside antibiotic. It would soon revolutionise the treatment of life-threatening diseases like tuberculosis.
Today, we have a deeper connection between deep-learning and antibiotics. In a December 2023 paper in Nature, scientists have reported discovering a new class of antibiotics using a form of deep-learning that has been gaining more attention.
According to their paper, the last known structural class of antibiotics was reported in 2000. Their work has thus ended a decades-long wait for a new class.
The researchers were from the Broad Institute, Integrated Biosciences Inc., the Wyss Institute for Biologically Inspired Engineering, and the Leibniz Institute of Polymer Research.
‘Enhancing drug development’
Unlike previous approaches that used deep-learning to discover new drugs, the researchers said they were able to identify the chemical motifs – technically called substructures – their model used to check whether a given compound could be an antibiotic. This, they wrote, made their model “explainable”.
The team also demonstrated the efficacy of two compounds from this novel class of antibiotics in mice infected with methicillin-resistant Staphylococcus aureus (MRSA). MRSA infections were responsible for more than 100,000 human deaths in 2019.
Amitesh Anand, a biologist who studies bacterial metabolism at the Tata Institute of Fundamental Research, Mumbai, lauded the study for advancing “our understanding in specific antibiotic research” and providing “a broader model for enhancing drug development strategies”.
AI researcher Vineeth Balasubramanian, a computer science and engineering professor at IIT Hyderabad, called the study “impressive”. “Being able to isolate substructures that have a certain tested property may be critical in scientific understanding as well as expediting drug discovery efforts,” he said.
Deep-learning, explain yourself
All artificial neural networks are made of artificial ‘neurons’. These are algorithms that receive an input, perform a computation, and relay the output. Deep-learning neural networks have three or more layers of such ‘neurons’.
Using these neural networks to make predictions has two steps: training and testing. In training, the network is provided with a large amount of annotated inputs. For example, if the network is being trained to identify pictures of cats, it is provided with many such pictures labelled “cat”.
During testing, the network is shown images outside the input dataset, e.g. one of a cat the network hasn’t encountered during training and another of a dog. If the network is able to accurately classify the former as ‘cat’ and the latter as ‘not cat’, the network is said to have ‘learnt’.
With most deep-learning networks, researchers have not been able to identify which aspects of the novel input the network used to make its evaluation. This renders most deep learning models a black box.
On the other hand, the network reported in the new study was explainable, which is the opposite. According to Prof. Balasubramanian, this would be like “a deep learning model predicting an image as that of a cat and then explaining which pixels in the image helped the model make this prediction”.
This is considered significant in antibiotic discovery. If deep-learning can identify potential antibiotics and also explain what substructures may contribute to its antibiotic activity, scientists can synthesise and test compounds with these substructures faster.
Predictions and rationales
Inspired to open up the ‘black box’, Felix Wong, a researcher at the Broad Institute, co-founder of Integrated Biosciences, and lead author of the paper, started by experimentally screening more than 39,000 compounds for their ability to inhibit the growth of S. aureus. These contained “most known antibiotics, natural products, and structurally diverse molecules,” the authors wrote. They finally shortlisted 512 compounds.
Then the team trained a graph neural network (GNN) on this dataset. A GNN represents atoms as “nodes” and the bonds between them as “edges” on a mathematical graph.
While searching for antibiotics, researchers are careful to choose compounds that don’t harm human cells. Testing the 512 active compounds on lab-grown human cells, the researchers found 306 compounds to fit this criterion. The team also trained other GNNs to identify cytotoxic compounds.
In the testing phase, the GNNs were exposed to a database of more than 1.2 crore compounds, and the networks identified 3,646 compounds that could be antibiotic.
The researchers hypothesised that the GNNs made their predictions based on a molecule’s substructures. (For example, several antibiotics have substructures called beta-lactam rings.) The team dubbed those substructures directly responsible for a compound’s antibiotic property ‘rationales’.
Using a different algorithm, the team determined the rationales of the 3,646 compounds – including previously known ones like beta-lactam rings, cephalosporin, and quinolone bicyclic rings.
Against MRSA and VRE
While determining the rationales for 380 compounds from the set of 3,646, the team found some that had not been previously reported and which the GNNs predicted could confer antibiotic properties to molecules. One such rationale was N-[2-(2-chlorophenoxy)ethyl]aniline (shown below). On further tests, two compounds containing this rationale were found to inhibit the growth of MRSA cultures by changing the concentration of hydrogen ions across the bacterial cell membrane.
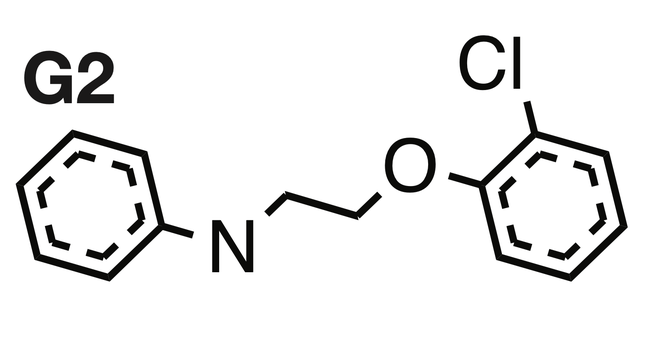
Notably, the compounds were also effective against vancomycin-resistant enterococci (VRE), a bacteria responsible for more than 5,400 deaths in the U.S. in 2017.
Finally, the team tested one compound in mouse models of MRSA-related skin and thigh infections and found that it effectively reduced the extent of infection.
The results mean the team can conduct more tests to ascertain their potential as antibiotics against MRSA. According to Prof. Balasubramanian, “there are many more studies and steps before a drug actually gets translated to use”.
A lacuna
The study’s key contribution lies in making deep-learning approaches to drug discovery explainable. This, Prof. Balasubramanian said, is significant “especially if the method is reproducible across drug categories”.
Dr. Wong said the team is now applying substructure rationales to design new antibiotics. It is also applying the approach to other drugs, including those that selectively kill ageing cells and thus prevent the onset of several age-related disorders.
Prof. Balasubramanian also flagged one lacuna in the new study: that the researchers had assessed the explainability of their system after they had predicted the antibiotic property of certain compounds.
As a result, any error in the explainability analysis would lead to “one never [knowing] whether the original deep-learning method focused on the wrong substructures or if the follow-up analysis was incorrect,” Prof. Balasubramanian explained.
As an alternative, he suggested the researchers could incorporate explainability implicitly in their deep-learning models – a direction that his group at IIT Hyderabad has been exploring. According to him, this would be akin to “teaching the model to learn to predict via reasoning, which is more robust”.
Sayantan Datta is a science journalist and a faculty member at Krea University. They tweet at @queersprings.