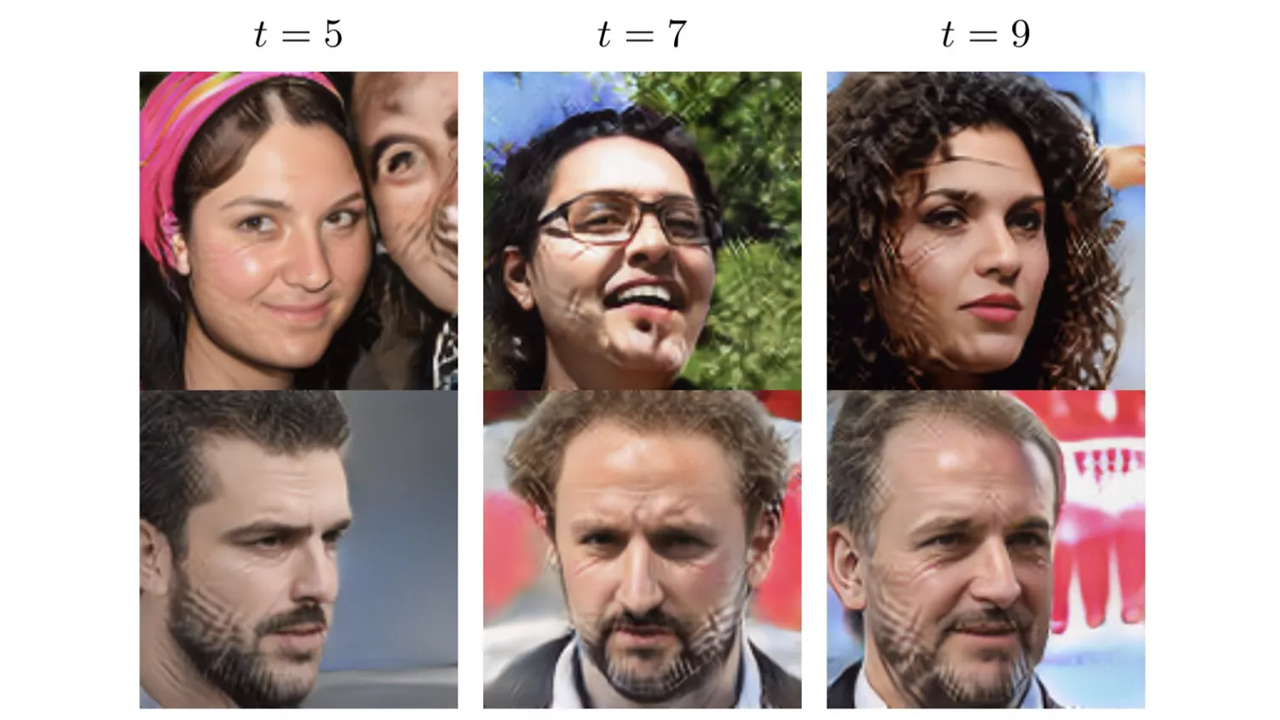
A new study on AI has found an inherent limitation on current-generation networks such as the ones employed by ChatGPT and Midjourney. It seems that AI networks trained on AI outputs (like the text created by ChatGPT or the image output created by a Stable Diffusion model) tend to go "MAD" after five training cycles with AI-generated data. As you can see in the above images, the result is oddly mutated outputs that aren't reflective of reality.
MAD - short for Model Autophagy Disorder — is the acronym used by the Rice and Stanford University researchers involved in the study to describe how AI models, and their output quality, collapses when repeatedly trained on AI-generated data. As the name implies, the model essentially "eats itself," not unlike the Ouroboros of myth. It loses information on the tails (the extremes) of the original data distribution, and starts outputting results that are more aligned with the mean representation of data, much like the snake devouring its own tail.
In work led by @iliaishacked we ask what happens as we train new generative models on data that is in part generated by previous models.We show that generative models lose information about the true distribution, with the model collapsing to the mean representation of data pic.twitter.com/OFJDZ4QofZJune 1, 2023
In essence, training an LLM on its own (or anothers') outputs creates a convergence effect on the data that composes the LLM itself. This can be easily seen in the graph above, shared by scientists and research team member Nicolas Papernot on Twitter, where successive training iterations on LLM-generated data leads the model to gradually (yet dramatically) lose access to the data contained at the extremities of the Bell curve - the outliers, the less common elements.
The data at the edges of the spectrum (that which has fewer variations and is less represented) essentially disappears. Because of that, the data that remains in the model is now less varied and regresses towards the mean. According to the results, it takes around five of these rounds until the tails of the original distribution disappear - that's the moment MAD sets in.
Cool paper from my friends at Rice. They look at what happens when you train generative models on their own outputs…over and over again. Image models survive 5 iterations before weird stuff happens.https://t.co/JWPyRwhW8oCredit: @SinaAlmd, @imtiazprio, @richbaraniuk pic.twitter.com/KPliZCABd4July 7, 2023
Model Autophagy Disorder isn't confirmed to affect all AI models, but the researchers did verify it against autoencoders, Gaussian mixture models, and large language models.
It just so happens that all of these types of models that can "go MAD" have been widespread and operating for a while now: autoencoders can handle things such as popularity prediction (in things such as a social media app's algorithm), image compression, image denoising, and image generation; and gaussian mixture models are used for density estimation, clustering, and image segmentation purposes, which makes them particularly useful for statistical and data sciences.
As for the large language models at the core of today's popular chatbot applications (of which OpenAI's ChatGPT and Anthropic's friendly AI Claude are mere examples), they, too, are prone to go MAD when trained on their own outputs. With that, it's perhaps worth stressing how important these AI systems are in our lives; algorithmic AI models are employed both in the corporate and public spheres.
We faced a similar issue while bootstrapping generative models for Sokoban level generation using https://t.co/ONWUSMnBTQOne solution was to cluster levels based on their characteristics and to change the batch sampling process to emphasize levels with rarer characteristics.June 6, 2023
This research provides a way to peer into the black box of AI development. And it shreds any hope that we'd found an endless fountain of data from making a hamster wheel out of certain AI models: feeding it data, and then feeding its own data back into it, in order to generate more data that's then fed back again.
This could be an issue for currently-existing models and applications of these models: if a model that's achieved commercial use has, in fact, been trained on its own outputs, then that model has likely regressed toward its mean (remember it takes around five cycles of input-output for that to manifest). And if that model has regressed towards its mean, then it's been biased in some way, shape, or form, as it doesn't consider the data that would naturally be in the minority. Algorithmic bigotry, if you will.
Another important point pushed forward by the results is the concern of data provenance: it now becomes even more important to be able to separate "original" data from "artificial" data. If you can't identify what data was created by an LLM or a generative image application, you might accidentally include it in training data for your next-generation product.
Unfortunately, that ship has already likely sailed: there's been a non-zero amount of unlabeled data that's been already produced by these types of networks and been incorporated into other systems. Even if we had a snapshot of the entire Internet before the popularity explosion for ChatGPT or Midjourney, there's long been AI-produced data poured daily onto the world wide web. And that's saying nothing of the immense amounts of data they've in the meantime produced.
But even if that ship has sailed, at least now we know. Knowing means that the search for a watermark that identifies AI-generated content (and that's infallible) has now become a much more important - and lucrative - endeavor, and that the responsibility for labeling AI-generated data has now become a much more serious requirement.
Apart from that, though, there are other ways to compensate for these biases. One of the ways is to simply change the model's weightings: if you increase how relevant or how frequent the results at the tails of the distribution are, they will naturally move along the bell curve, closer to the mean. It follows that they would then be much less prone to "pruning" from the self-generative training: the model still loses the data at the edges of the curve, but that data is no longer only there.
But then, how is the weighting decided? In what measure should the weightings be moved? The frequency increased? There's also a responsibility here to understand the effects of model fine-tuning and how those impact the output as well.
For each question that's answered, there are a number of others that jump to the foreground: questions relating to the truth behind the model's answers (where deviations are known as hallucinations); whether or not the model is biased, and where does this bias come from (if from the training data itself or from the weighting process used to create the network, and now we know from the MAD process as well); and of course, what happens when models are trained on their own data. And as we've seen, the results aren't virtuous.
And they couldn't be: people with no access to new experiences wither too, so they become echo chambers of what has come before. And that's exactly the same as saying something along the lines of "when the model is trained on its own outputs, it collapses."