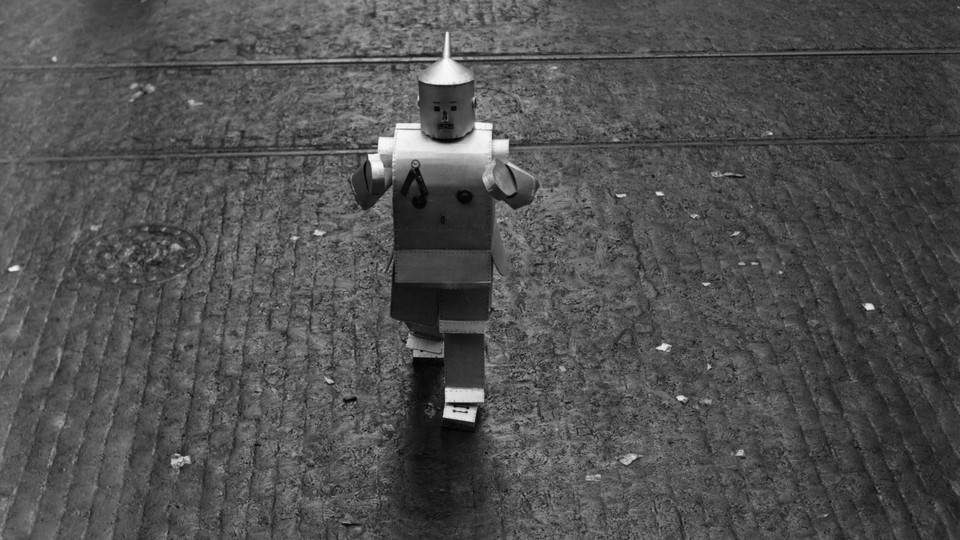
Last week, Google put one of its engineers on administrative leave after he claimed to have encountered machine sentience on a dialogue agent named LaMDA. Because machine sentience is a staple of the movies, and because the dream of artificial personhood is as old as science itself, the story went viral, gathering far more attention than pretty much any story about natural-language processing (NLP) has ever received. That’s a shame. The notion that LaMDA is sentient is nonsense: LaMDA is no more conscious than a pocket calculator. More importantly, the silly fantasy of machine sentience has once again been allowed to dominate the artificial-intelligence conversation when much stranger and richer, and more potentially dangerous and beautiful, developments are under way.
The fact that LaMDA in particular has been the center of attention is, frankly, a little quaint. LaMDA is a dialogue agent. The purpose of dialogue agents is to convince you that you are talking with a person. Utterly convincing chatbots are far from groundbreaking tech at this point. Programs such as Project December are already capable of re-creating dead loved ones using NLP. But those simulations are no more alive than a photograph of your dead great-grandfather is.
Already, models exist that are more powerful and mystifying than LaMDA. LaMDA operates on up to 137 billion parameters, which are, speaking broadly, the patterns in language that a transformer-based NLP uses to create meaningful text prediction. Recently I spoke with the engineers who worked on Google’s latest language model, PaLM, which has 540 billion parameters and is capable of hundreds of separate tasks without being specifically trained to do them. It is a true artificial general intelligence, insofar as it can apply itself to different intellectual tasks without specific training “out of the box,” as it were.
Some of these tasks are obviously useful and potentially transformative. According to the engineers—and, to be clear, I did not see PaLM in action myself, because it is not a product—if you ask it a question in Bengali, it can answer in both Bengali and English. If you ask it to translate a piece of code from C to Python, it can do so. It can summarize text. It can explain jokes. Then there’s the function that has startled its own developers, and which requires a certain distance and intellectual coolness not to freak out over. PaLM can reason. Or, to be more precise—and precision very much matters here—PaLM can perform reason.
The method by which PaLM reasons is called “chain-of-thought prompting.” Sharan Narang, one of the engineers leading the development of PaLM, told me that large language models have never been very good at making logical leaps unless explicitly trained to do so. Giving a large language model the answer to a math problem and then asking it to replicate the means of solving that math problem tends not to work. But in chain-of-thought prompting, you explain the method of getting the answer instead of giving the answer itself. The approach is closer to teaching children than programming machines. “If you just told them the answer is 11, they would be confused. But if you broke it down, they do better,” Narang said.
Google illustrates the process in the following image:
Adding to the general weirdness of this property is the fact that Google’s engineers themselves do not understand how or why PaLM is capable of this function. The difference between PaLM and other models could be the brute computational power at play. It could be the fact that only 78 percent of the language PaLM was trained on is English, thus broadening the meanings available to PaLM as opposed to other large language models, such as GPT-3. Or it could be the fact that the engineers changed the way that they tokenize mathematical data in the inputs. The engineers have their guesses, but they themselves don’t feel that their guesses are better than anybody else’s. Put simply, PaLM “has demonstrated capabilities that we have not seen before,” Aakanksha Chowdhery, a member of the PaLM team who is as close as any engineer to understanding PaLM, told me.
None of this has anything to do with artificial consciousness, of course. “I don’t anthropomorphize,” Chowdhery said bluntly. “We are simply predicting language.” Artificial consciousness is a remote dream that remains firmly entrenched in science fiction, because we have no idea what human consciousness is; there is no functioning falsifiable thesis of consciousness, just a bunch of vague notions. And if there is no way to test for consciousness, there is no way to program it. You can ask an algorithm to do only what you tell it to do. All that we can come up with to compare machines with humans are little games, such as Turing’s imitation game, that ultimately prove nothing.
Where we’ve arrived instead is somewhere more foreign than artificial consciousness. In a strange way, a program like PaLM would be easier to comprehend if it simply were sentient. We at least know what the experience of consciousness entails. All of PaLM’s functions that I’ve described so far come from nothing more than text prediction. What word makes sense next? That’s it. That’s all. Why would that function result in such enormous leaps in the capacity to make meaning? This technology works by substrata that underlie not just all language but all meaning (or is there a difference?), and these substrata are fundamentally mysterious. PaLM may possess modalities that transcend our understanding. What does PaLM understand that we don’t know how to ask it?
Using a word like understand is fraught at this juncture. One problem in grappling with the reality of NLP is the AI-hype machine, which, like everything in Silicon Valley, oversells itself. Google, in its promotional materials, claims that PaLM demonstrates “impressive natural language understanding.” But what does the word understanding mean in this context? I am of two minds myself: On the one hand, PaLM and other large language models are capable of understanding in the sense that if you tell them something, its meaning registers. On the other hand, this is nothing at all like human understanding. “I find our language is not good at expressing these things,” Zoubin Ghahramani, the vice president of research at Google, told me. “We have words for mapping meaning between sentences and objects, and the words that we use are words like understanding. The problem is that, in a narrow sense, you could say these systems understand just like a calculator understands addition, and in a deeper sense they don’t understand. We have to take these words with a grain of salt.” Needless to say, Twitter conversations and the viral information network in general are not particularly good at taking things with a grain of salt.
Ghahramani is enthusiastic about the unsettling unknown of all of this. He has been working in artificial intelligence for 30 years, but told me that right now is “the most exciting time to be in the field” exactly because of “the rate at which we are surprised by the technology.” He sees huge potential for AI as a tool in use cases where humans are frankly very bad at things but computers and AI systems are very good at them. “We tend to think about intelligence in a very human-centric way, and that leads us to all sorts of problems,” Ghahramani said. “One is that we anthropomorphize technologies that are dumb statistical-pattern matchers. Another problem is we gravitate towards trying to mimic human abilities rather than complementing human abilities.” Humans are not built to find the meaning in genomic sequences, for example, but large language models may be. Large language models can find meaning in places where we can find only chaos.
Even so, enormous social and political dangers are at play here, alongside still hard-to-fathom possibilities for beauty. Large language models do not produce consciousness but they do produce convincing imitations of consciousness, which are only going to improve drastically, and will continue to confuse people. When even a Google engineer can’t tell the difference between a dialogue agent and a real person, what hope is there going to be when this stuff reaches the general public? Unlike machine sentience, these questions are real. Answering them will require unprecedented collaboration between humanists and technologists. The very nature of meaning is at stake.
So, no, Google does not have an artificial consciousness. Instead, it is building enormously powerful large language systems with the ultimate goal, as Narang said, “to enable one model that can generalize across millions of tasks and ingest data across multiple modalities.” Frankly, it’s enough to worry about without the science-fiction robots playing on the screens in our head. Google has no plans to turn PaLM into a product. “We shouldn’t get ahead of ourselves in terms of the capabilities,” Ghahramani said. “We need to approach all of this technology in a cautious and skeptical way.” Artificial intelligence, particularly the AI derived from deep learning, tends to rise rapidly through periods of shocking development, and then stall out. (See self-driving cars, medical imaging, etc.) When the leaps come, though, they come hard and fast and in unexpected ways. Gharamani told me that we need to achieve these leaps safely. He’s right. We’re talking about a generalized-meaning machine here: It would be good to be careful.
The fantasy of sentience through artificial intelligence is not just wrong; it’s boring. It’s the dream of innovation by way of received ideas, the future for people whose minds never escaped the spell of 1930s science-fiction serials. The questions forced on us by the latest AI technology are the most profound and the most simple; they are questions that, as ever, we are completely unprepared to face. I worry that human beings may simply not have the intelligence to deal with the fallout from artificial intelligence. The line between our language and the language of the machines is blurring, and our capacity to understand the distinction is dissolving inside the blur.