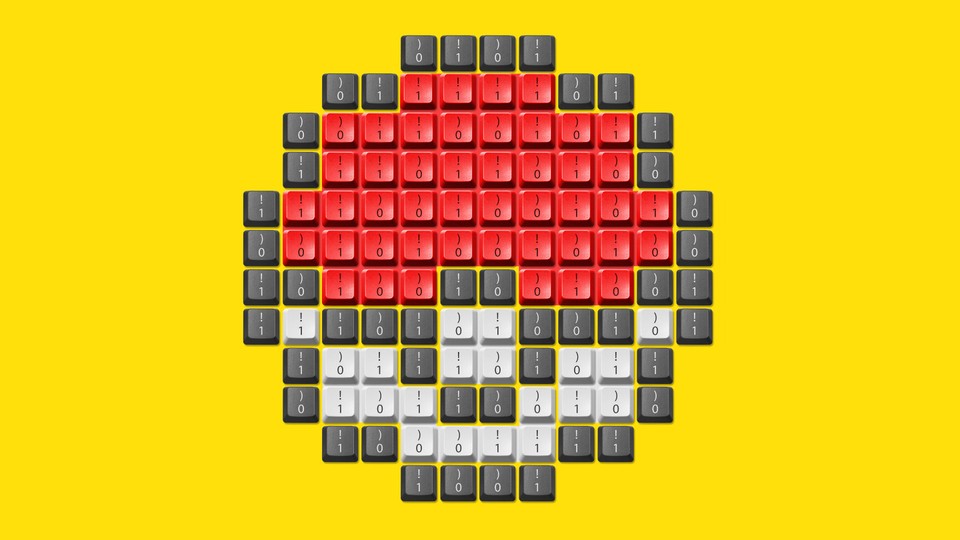
For a brief moment, we could taste victory. On the first turn of our online Pokémon battle against the player Athena2023, our Aegislash dealt some serious damage to Athena2023’s Gengar. One more blow like that, and Gengar, a little purple ghost with a sinister grin, would be toast.
But Athena2023 had other ideas. Before we could make another move, Gengar blew away Aegislash, a levitating Pokémon with the appearance of a zoomorphic sword and shield, with a single attack. The point of a battle is to use your team of six Pokémon to knock out all six of your opponent’s, and we were losing fast. Athena2023 sent out a Lunala, a demonic purple-and-white bird with scythes for wings, which proceeded to eviscerate three straight Pokémon in an attack called Moongeist Beam. By turn 13, we had lost four of our Pokémon and defeated exactly zero of Athena2023’s.
Such a pathetic showing might ordinarily elicit a smirk or some pity from an opposing player. But Athena2023 is not capable of smirking or extending pity—or of anything other than playing Pokémon, for that matter—because it is an AI designed by the computer scientist Nicholas Sarantinos. Nor, it turned out, did we have much to be ashamed of: Athena2023 had at one point ranked 33rd in the world on the online battle simulator Pokémon Showdown.
Teaching an AI to play Pokémon is pretty impressive—and perhaps a tad frivolous. But Sarantinos envisioned his program as more than a way to wreck mediocre players like us. In his paper, which has yet to be peer-reviewed, he writes that it might “inspire all sorts of applications that require team management under conditions of extreme uncertainty,” such as a team of doctors in “a pandemic stricken region or a war-zone.” That seems like a leap, but game-play has for decades provided a way to experiment with AI not yet ready for the real world. What, after all, is life if not the most complicated game of all?
In a sense, the most surprising thing about Pokémon AIs is not that they exist but that they did not exist sooner. Back in the 1950s, some of the first rudimentary AIs were chess- and checkers-playing programs. The idea was straightforward, Julian Togelius, an NYU computer scientist who has written extensively on AI and games, told us: “We want to create artificial intelligence, so let’s do the things smart people do.” In 1997, IBM’s Deep Blue supercomputer defeated the world chess champion Garry Kasparov, and a decade later, checkers was “solved” entirely. In recent years, AI researchers have branched out to a far wider variety of games. Their machines have played poker, Pac-Man, and Super Mario Bros. The pursuit is not fringe: In just the past few months, the AI powerhouses Meta and Deepmind have published new work on Stratego, Diplomacy, and Minecraft.
Mastering any of these is challenging—but that it’s possible at all makes games an attractive target for programmers. “Games have been the core test bed of AI investigations,” says Georgios Yannakakis, the director of the Institute of Digital Games at the University of Malta. They are controllable in a way that real life isn’t: A game of chess unfolds on 64 squares, uses six types of pieces that make only certain moves, and always ends in a win, draw, or loss. More complex games, whether with a large three-dimensional world like Minecraft or hidden information like poker, still have rules and outcomes. Even a virtual road designed to train driving AI is, in this sense, a game.
In games and simulations, researchers can access huge amounts of data, break things for free, and easily determine their software’s success. Once developed in a game, an algorithm might be applicable elsewhere—research on chess and the Chinese strategy game Go has advanced numerous state-of-the-art AI algorithms; simulated game-like environments are helping AI navigate 3-D space; poker has improved computers’ ability to reason with imperfect information.
But the very reasons games are attractive test beds are precisely why they can hinder research: Controlled environments, clear benchmarks, and established rules, even in complex and three-dimensional games, are “in some ways idealizations of real life,” says Melanie Mitchell, who studies natural and artificial intelligence at the Santa Fe Institute. Skills that go into winning a game don’t easily transfer to, or might even disguise challenges arising in, the much more complex real world. A human who exclusively played chess wouldn’t be able to do much else; that’s not how most people live, but it’s precisely how computers work. Indeed, decades of work on chess-playing programs, though fruitful, have also bent AI research toward specific game-playing techniques at the expense of other real-world approaches.
But a clear real-world application might be of secondary concern for some programmers—a computer crushing humans at any game makes for fantastic press. “You have a large number of researchers and research funding really focused on making really nice demos,” says Deborah Raji, an AI researcher and a fellow at Mozilla, “and not really yielding meaningful progress on real-world problems.” Self-driving cars, after seamless test runs, crash constantly. Even language models such as the one underlying ChatGPT, with a singular focus on the “game” of text prediction, prove terrible in other domains.
Or perhaps expecting an algorithm to transfer seamlessly from game to world is itself a simplification of discovery—much of the research in not just computer science but also number theory, biology, and really any field lies dormant before resurfacing in unexpected ways. Penicillin and Coca-Cola were accidents; after frustrating mathematicians for three centuries, Fermat’s Last Theorem was solved by proving a seemingly unrelated conjecture. “In two, three years time, there might be someone who takes the algorithm [from a game] and applies it to something completely different, and we have a breakthrough,” Yannakakis said.
Arguably the most impressive recent game-playing AI is Cicero, designed last year by a team of researchers at Meta to play Diplomacy, a strategic board game that is sort of like European, World War I–era Risk, only without the elements of chance and with far more negotiation, cooperation, and interpersonal maneuvering. Like ChatGPT, Cicero relies on a language model to communicate with its human opponents, but unlike ChatGPT, it must connect those words with actions. It must have a theory of mind. “We were actually trying to brainstorm what would be the hardest game to make an AI for,” Noam Brown, one of the Meta researchers who designed Cicero, told us.
But an even harder challenge might be designing AIs that can play multiple games. Some of these already exist: Deepmind’s MuZero is proficient at chess, Go, shogi (also known as Japanese chess), and 57 different Atari games. The greater the number and diversity of the set of games, the more difficult it is to design a single AI capable of playing them all. “If we had a piece of software that could just take any game from the top 200 list on the App Store … and play it as well as a good human, would we have artificial [general] intelligence? We would have something like it,” Togelius said. Add up all the games, and eventually you get something like life.
That potential is what drew Sarantinos to Pokémon: The game seemed to present a number of interesting challenges, with its complicated rules, incomplete information, and pure stochasticity, not to mention the brain-breaking number of possible ways a battle can play out. The connection between managing six digital Pokémon in an online battle and managing six human doctors in an actual war zone seems, it must be said, rather tenuous. But Sarantinos’s real hope, he said, is that his work will serve as a benchmark against which future, more advanced AIs can be tested.
If those more advanced agents are even stronger Pokémon players than Athena2023, then we want nothing to do with them. By the time we finally managed to defeat one of Athena’s six Pokémon, just one of our own remained—Hitmonlee, a neckless kickboxing Pokémon with no apparent mouth, nose, or ears. Back came Lunala, the creepy bird-scythe hybrid that had so summarily dispatched half of our team. Lunala’s particular abilities make it entirely impervious to all of Hitmonlee’s attacks, such that Athena2023 could have finished off Hitmonlee—and the battle—with a single strike. And yet, it did not.
Instead, strangely, it used a move that does no damage to the opponent. Then it used the move again … and again … and again and again and again. On the threshold of victory, Athena2023 made the same pointless move 12 consecutive times. Had we not known better, we might have thought it was mocking us. But this was sheer idiocy, proof that although Athena2023 was more than intelligent enough to win its game, it was still not very intelligent at all. The AI was not unlike our pitiful Hitmonlee: great at kickboxing opponents to death but also neckless, mouthless, noseless, earless, brainless.