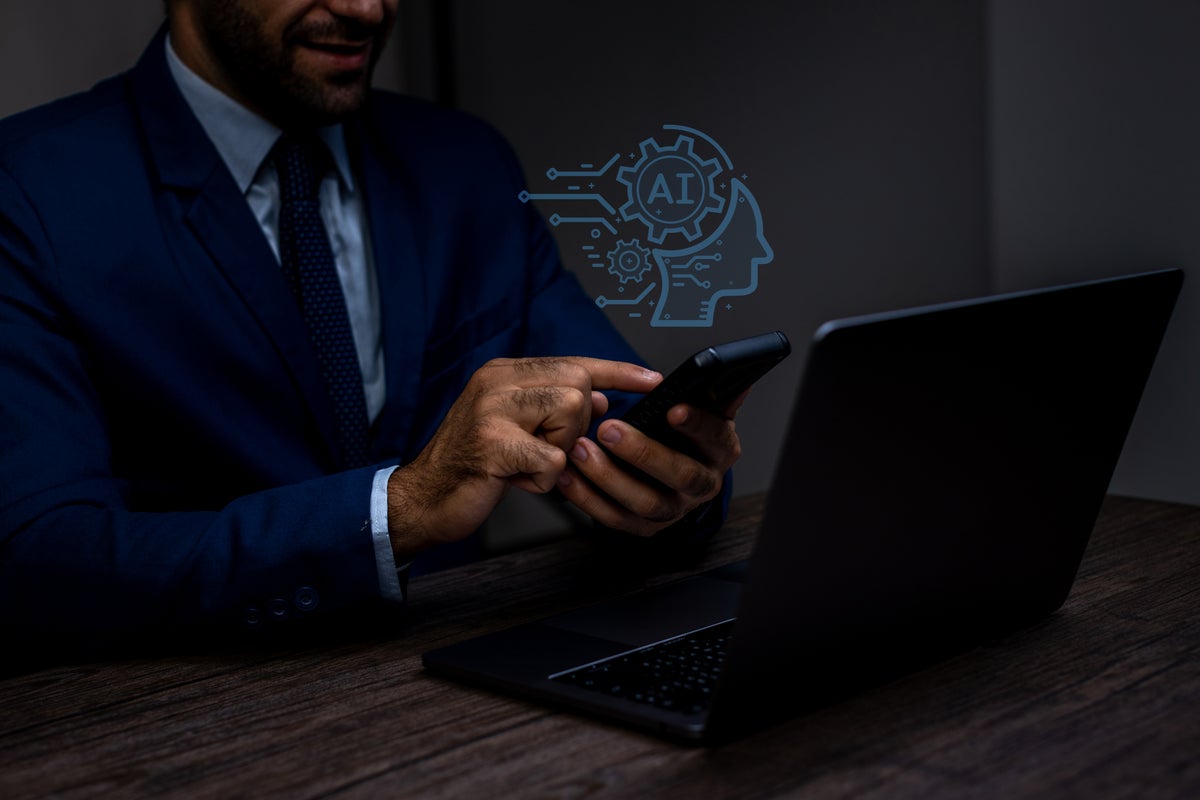
Fivetran is a Business Reporter client.
From ride-hailing apps to CCTV cameras and everyday technologies enhanced with advanced pattern-recognition and machine learning (ML) capabilities to improve experiences, AI is already everywhere.
It’s also the next frontier in unlocking business agility and innovation. But AI is only as good as the data it consumes – and with the dawn of large language models (LLMs) and tools such as ChatGPT accelerating AI adoption, businesses face increased pressure to ensure bad data is not eating into their competitive edge.
The problem is, most organisations still battle widespread data quality issues that hinder these advancements – and 87 per cent of them believe that those that fail to embrace AI will fall by the wayside. In fact, nine in ten organisations still lack the kind of automation capabilities that would enable clean and timely data to be fed to vital ML programmes. These were key findings in Fivetran’s recent AI research.
What generative AI is, and what it isn’t
Although generative AI tools may seem intuitive, education must come before adoption. Businesses need to be particularly aware of generative AI’s limitations, and our human biases, such as the susceptibility to perceive human-like intelligence in places where it doesn’t exist.
AI is defined by ultra-fast processing power and a deeply intricate understanding of associations. Without the context of human existence, LLMs only have data to rely on. So, the first question business leaders need to ask to get started with any type of AI is whether they trust the data itself.
Data is at the heart of AI success
AI outcomes will only ever be as trustworthy as the data fed to them. Flawed data processes will be more apparent in generative AI use than in most other areas, but while humans can separate sense from nonsense, machines can’t – potentially leading to misguided business decision-making.
Fivetran’s research shows that senior technical stakeholders don’t trust AI – and, in fact, are losing revenues due to underperforming AI models based on bad data. Yet when asked about the effectiveness of their underlying data processes, the most common issues cited are some of the most fundamental: inaccessible data and top talent bogged down in manual and repetitive tasks.
To successfully use data in a generative AI context, businesses must make vital data accessible, while creating a strong governance framework for what data can be included in analysis, how it can be processed and how it can be accessed.
Making data accessible
A lack of access to timely and relevant data is one of the biggest growth-inhibitors for data teams. Data engineers, analysts and scientists all waste vast resources each day trying to manually unearth the data they need – by which time, insights are often outdated. Data analysts alone estimate they lose a third of every workday to ineffectual data processes. If top talent struggles to make data work, what chance do large language models have?
Automation is key to solving the data reliability challenge. Automating data movement can provide real-time access to all of an organisation’s data, regardless of which department created it or whether it resides in software applications or on-premises databases. This way, businesses can ensure that all employees and AI models use only the freshest data, and consequently, that all outcomes are also trustworthy. Better still, through automation, they can predefine the rules of this data use.
Enforce good data governance
The same way you wouldn’t give every visitor to your home the code to your safe, so must there be limitations on what data businesses give AI access to. Businesses have a responsibility to safeguard sensitive data such as personally identifiable information (PII) and mask these when data sets are used for analysis. They must also be able to track the lineage of data: which system accessed what data, when and how. This is metadata – the data about data.
This level of visibility and control over data use is crucial if organisations are to establish the watertight data governance policies and processes that regulators increasingly expect and customers outright demand. Luckily, technology already provides an answer to these modern woes. With metadata sharing and integration with automation tools, businesses don’t need to start from scratch to take advantage of the AI revolution.
Is your data ready to do the heavy-lifting?
Generative AI is on a meteoric rise and it seems every business wants a slice of the action. But amid this rapid adoption there is also mounting pressure on organisations to use new tools responsively and in a way that helps humans do their jobs more effectively. Organisations that already take advantage of automated data movement and solid data governance foundations won’t need to apply the brakes on AI projects. For others, the age-old wisdom holds: run before you walk and bolster your data strategy first – then your business will be ready to make strides in its generative AI journey.